The Controversy Surrounding Google DeepMind's AlphaChip Claims
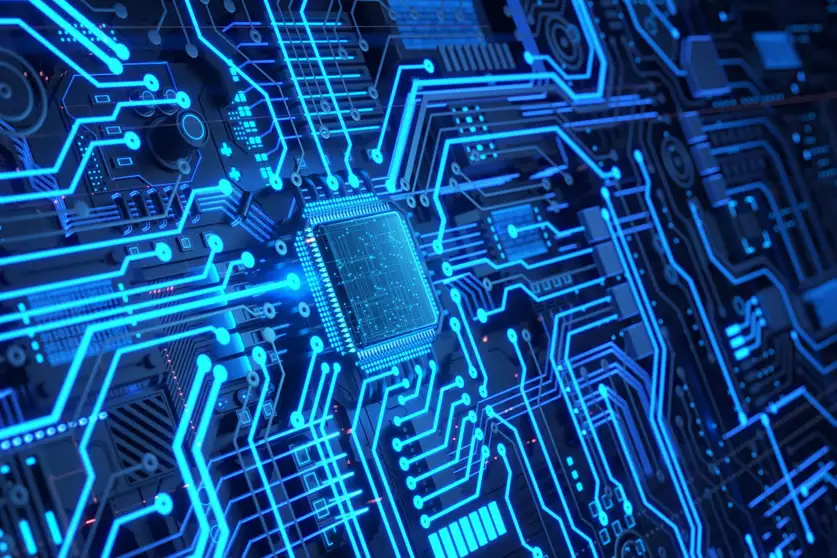
Google DeepMind asserts that its AI technology, AlphaChip, is capable of designing chip layouts that surpass human abilities and is already in use within its data centers and smartphones. However, some experts in chip design express skepticism regarding these claims, highlighting a lack of public evidence to substantiate them.
According to researchers Anna Goldie and Azalia Mirhoseini, the AlphaChip approach can produce “superhuman chip layouts” in mere hours, a process that traditionally takes weeks or months of human labor. The AI utilizes reinforcement learning to understand the interconnections among various chip components, receiving rewards based on the quality of the final layout. Yet, independent experts argue that the company has yet to demonstrate that AlphaChip consistently outperforms skilled human designers or existing commercial software, insisting on the need for public benchmarks that reflect current circuit design standards.
Patrick Madden from Binghamton University notes that if Google were to release experimental results for their designs, it would facilitate meaningful comparisons, which he believes the scientific community would readily accept. He points out that such experiments could be conducted in a matter of days, especially given Google's extensive resources. The absence of these results raises questions about the validity of their claims.
Google DeepMind recently updated its 2021 paper published in Nature, stating that AlphaChip has contributed to the design of multiple generations of Tensor Processing Units (TPUs), specialized chips integral for training generative AI models, including Google’s Gemini chatbot. The company asserts that these AI-generated chip designs not only perform better than those crafted by human experts but are also steadily improving. The efficiency of AlphaChip lies in its ability to minimize the wiring needed to connect chip components, which can enhance processing speed and reduce power consumption. Moreover, AlphaChip has purportedly assisted in creating general-purpose chips for Google’s data centers and contributed to MediaTek's chip development for Samsung devices.
However, critics like Igor Markov from a rival firm point out that the publicly available code lacks compatibility with standard industry chip data formats, indicating that AlphaChip may currently be tailored for Google’s proprietary needs. He emphasizes that the true capabilities of AlphaChip remain unclear, noting that reinforcement learning typically demands significantly more computational resources than conventional commercial methods, often resulting in inferior outcomes.
Both Markov and Madden challenge the initial paper’s assertions about AlphaChip's superiority over unspecified human designers. They argue that comparisons to unnamed experts lack objectivity, reproducibility, and scientific merit. Madden likens the situation to AlphaGo claiming victories over unverified Go players, emphasizing the need for clear, verifiable evidence.
In a recent turn of events, an independent expert, Andrew Kahng from UC San Diego, withdrew his earlier praise for Google’s work after conducting a benchmarking effort that failed to confirm the AI's claims. Kahng’s findings indicated that AlphaChip did not consistently outperform a skilled human expert or established commercial algorithms, with the top-performing methods often being tools from companies like Cadence and NVIDIA.
Madden concludes that, based on fair comparisons, reinforcement learning appears to fall short of state-of-the-art performance in circuit design, raising doubts about its viability as a promising research avenue in the field.